Case Study
Home » Cybersecurity Threat Detection
Cybersecurity Threat Detection
Problem Statement
Organizations face an increasing number of sophisticated cybersecurity threats, including malware, phishing attacks, and unauthorized access. A financial institution experienced frequent attempts to breach its network, risking sensitive data and regulatory compliance. Traditional security measures were reactive and failed to detect threats in real time. The institution sought a proactive AI-driven solution to identify and prevent cybersecurity threats effectively.
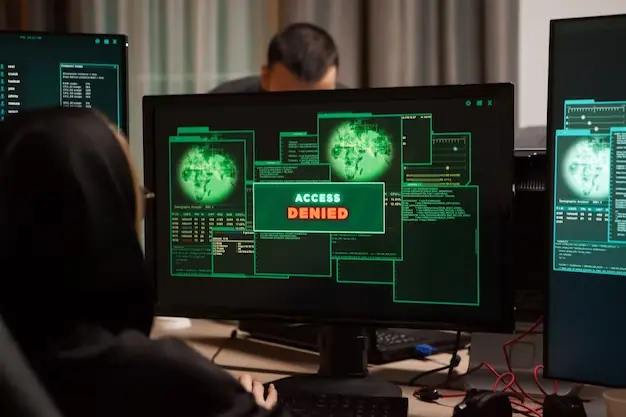
Challenge
Developing an advanced threat detection system required addressing several challenges:
- Processing and analyzing large volumes of network traffic and user activity data in real time.
- Identifying new and evolving threats, such as zero-day vulnerabilities, with high accuracy.
Minimizing false positives to ensure security teams could focus on genuine threats.
Solution Provided
An AI-powered threat detection system was developed using machine learning algorithms and advanced analytics. The solution was designed to:
- Continuously monitor network activity and user behavior to identify suspicious patterns.
- Detect and neutralize cybersecurity threats in real time, including malware and phishing attempts.
- Provide actionable insights to security teams for faster and more effective threat response.
Development Steps

Data Collection
Collected network traffic logs, endpoint activity, and historical threat data to train machine learning models.

Preprocessing
Cleaned and standardized data, ensuring compatibility across diverse sources, and filtered out noise for accurate analysis.

Model Development
Developed machine learning algorithms for anomaly detection, behavioral analysis, and threat classification. Trained models on labeled datasets to recognize known threats and identify emerging attack patterns.

Validation
Tested the system against simulated and real-world threat scenarios to evaluate detection accuracy, response times, and reliability.

Deployment
Integrated the threat detection system into the institution’s existing cybersecurity infrastructure, including firewalls, SIEM (Security Information and Event Management) tools, and endpoint protection.

Continuous Monitoring & Improvement
Established a feedback loop to refine models using new threat data and adapt to evolving attack strategies.
Results

Enhanced Security Posture
The system improved the institution’s ability to detect and prevent cybersecurity threats proactively, strengthening its overall security framework.

Reduced Incidence of Cyber Attacks
Real-time detection and response significantly reduced the frequency and impact of successful cyber attacks.

Improved Threat Response Times
Automated threat identification and prioritization enabled security teams to respond faster and more effectively to potential breaches.

Minimized False Positives
Advanced algorithms reduced false alarms, allowing security teams to focus on genuine threats and improve efficiency.

Scalable and Adaptive Solution
The system adapted to new threats and scaled effortlessly to protect growing organizational networks and data.