Case Study
Home » AI-Driven Hyper-Personalization
AI-Driven Hyper-Personalization
Problem Statement
The e-commerce industry struggles to retain customers and boost sales in a highly competitive market where generic marketing and product recommendations fail to meet individual expectations. A leading online retailer aimed to implement AI-driven hyper-personalization to deliver ultra-tailored shopping experiences, increase customer engagement, and maximize revenue through real-time, individualized strategies.
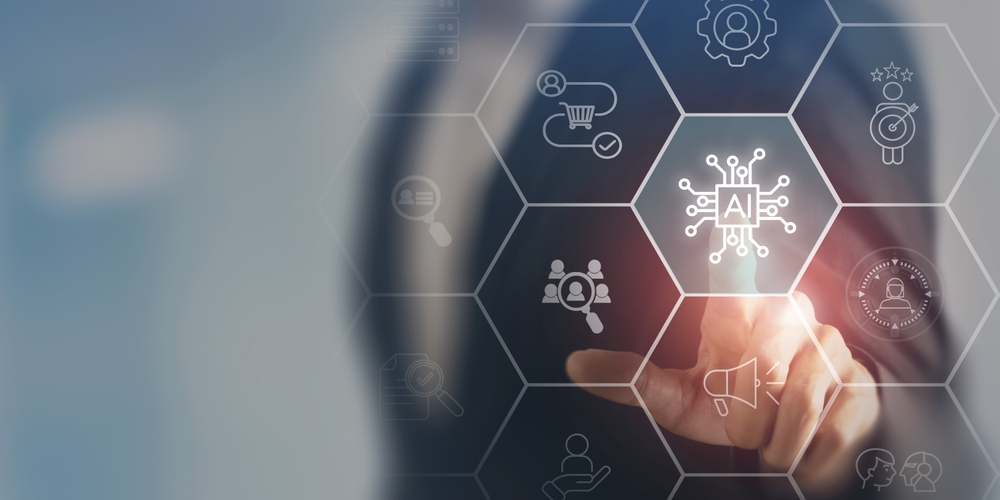
Challenge
The primary challenges in deploying AI-driven hyper-personalization included:
- Data Volume and Variety: Processing massive, diverse datasets—such as browsing history, purchase records, and social media activity—in real time to understand customer preferences.
- Real-Time Adaptation: Ensuring AI models could dynamically adjust recommendations and pricing for each user without latency or disruption.
- Customer Trust: Balancing personalization with privacy concerns to maintain user confidence while delivering relevant experiences.
Solution Provided
The solution leveraged advanced AI models to analyze customer data and deliver hyper-personalized shopping experiences. The system was designed to:
- Analyze Behavior: Use machine learning to interpret customer interactions, preferences, and intent across multiple touchpoints.
- Tailor Experiences: Generate real-time product recommendations, personalized discounts, and dynamic website content unique to each user.
- Optimize Revenue: Implement adaptive pricing and promotions based on individual purchasing patterns and market trends.
Development Steps

Data Collection
Aggregated data from customer profiles, website interactions, mobile app usage, and third-party sources like social media and loyalty programs.

Preprocessing
Cleaned and enriched the data, resolving inconsistencies and creating unified customer profiles for analysis.

Model Development
Developed a deep learning model (e.g., a recommendation system with collaborative filtering and reinforcement learning) to predict preferences and optimize personalization in real time.

Validation
Tested the model’s effectiveness using metrics like click-through rates, conversion rates, and customer satisfaction, refining it based on A/B testing results.

Deployment
Integrated the AI system into the retailer’s e-commerce platform, enabling dynamic updates to product displays, emails, and pricing during user sessions.

Continuous Monitoring & Improvement
Monitored user engagement and sales data, updating the model with new behavioral inputs to refine personalization over time.
Results

Increased Conversion Rates
Hyper-personalized recommendations boosted conversion rates by 28%, driving higher sales per visit.

Enhanced Customer Engagement
Tailored experiences increased average session time by 35%, reflecting deeper user interaction with the platform.

Higher Revenue
Dynamic pricing and promotions lifted average order value by 18%, optimizing profitability.

Improved Retention
Personalized follow-ups and offers reduced churn rates by 15%, strengthening customer loyalty.

Scalable Personalization
The AI system successfully scaled to handle millions of users simultaneously, maintaining performance during peak shopping seasons.