Case Study
Home » AI for Dynamic Pricing in Retail
AI for Dynamic Pricing in Retail
Problem Statement
Retail businesses face intense pressure to balance competitive pricing with profitability in a fast-paced, demand-driven market. Our client, a multinational e-commerce retailer, sought to utilize AI modeling to optimize their pricing strategy dynamically, ensuring they could respond to market trends, customer behavior, and competitor actions in real time while boosting revenue and maintaining customer loyalty.
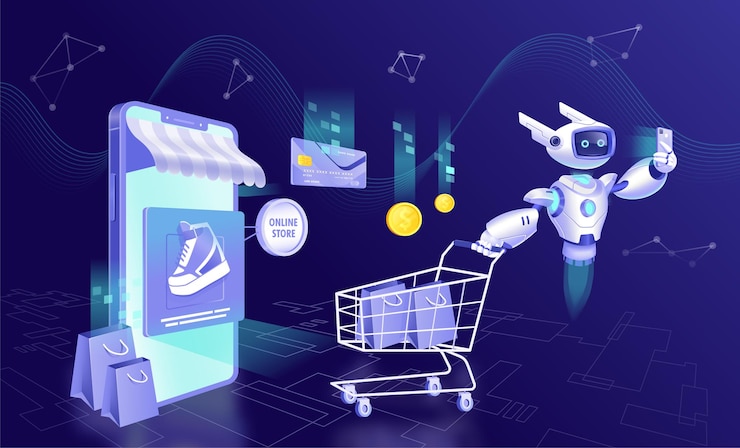
Challenge
The primary challenges in deploying an AI-driven dynamic pricing model included:
- Data Diversity: Processing vast amounts of heterogeneous data, including customer purchase history, competitor pricing, inventory levels, and market trends.
- Pricing Accuracy: Developing a model that accurately adjusts prices to maximize revenue without alienating price-sensitive customers.
System Integration: Seamlessly embedding the pricing model into the client’s e-commerce platform for real-time updates without disrupting operations
Solution Provided
The solution leveraged machine learning and real-time analytics to create a dynamic pricing optimization system. The system was designed to:
- Analyze Market Signals: Evaluate customer behavior, demand fluctuations, competitor pricing, and inventory data to inform pricing decisions.
- Optimize Prices Dynamically: Adjust product prices in real time to balance profitability and competitiveness while preserving customer trust.
Personalize Offers: Deliver tailored discounts and promotions to specific customer segments, enhancing loyalty and conversion rates.
Development Steps

Data Collection
Gathered comprehensive datasets, including historical sales, competitor pricing, customer demographics, and external market indicators

Preprocessing
Cleaned and standardized data, handling inconsistencies, normalizing inputs, and preparing time-series data for analysis.

Model Development
Trained machine learning models using regression algorithms and reinforcement learning to predict optimal price points based on demand and competition.

Validation
Tested the model’s performance with metrics like revenue uplift, conversion rates, and customer retention, refining it through A/B testing.

Deployment
Integrated the pricing model into the client’s e-commerce platform, enabling real-time price adjustments and personalized offers.

Continuous Monitoring & Improvement
Monitored pricing effectiveness and updated the model with fresh data to adapt to evolving market conditions and customer preferences.
Results

30% Revenue Increase
Dynamic pricing maximized profitability by aligning prices with demand and competition, boosting overall revenue.

15% Higher Conversion Rates
Personalized offers and competitive pricing drove more purchases, especially among price-sensitive customers.

20% Improved Inventory Turnover
Optimized pricing reduced excess stock, streamlining supply chain operations.

Enhanced Customer Loyalty
Tailored promotions increased repeat purchases by 12%, strengthening brand affinity.

Scalable Pricing Strategy
The system handled a 40% surge in traffic during peak sales seasons without performance issues.