Case Study
Home » AI for Sustainability and Climate Modeling
AI for Sustainability and Climate Modeling
Problem Statement
The renewable energy sector faces challenges in maximizing efficiency and minimizing environmental impact due to unpredictable weather patterns, fluctuating energy demand, and complex grid management. A leading renewable energy company aimed to leverage AI for sustainability and climate modeling to optimize solar and wind energy production, reduce carbon emissions, and enhance grid reliability, contributing to global sustainability goals.
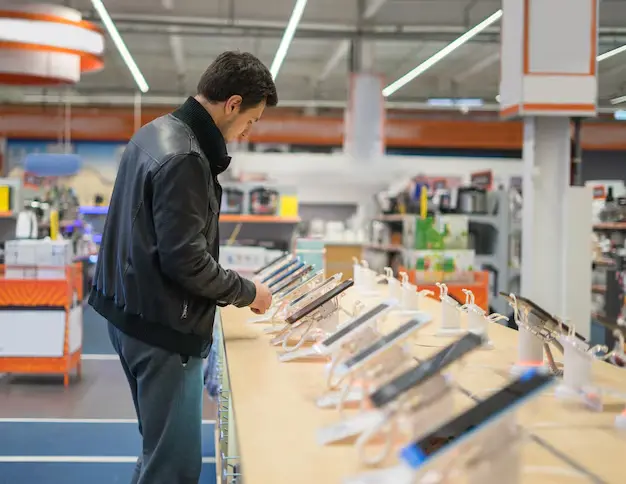
Challenge
The key challenges in implementing AI for this purpose included:
- Data Complexity: Integrating and analyzing vast datasets from weather forecasts, energy production logs, and grid performance metrics, often in real time.
- Predictive Precision: Developing accurate models to forecast weather patterns and energy output, critical for balancing supply and demand.
- System Integration: Embedding AI solutions into existing energy infrastructure to enable actionable insights without disrupting operations.
Solution Provided
The solution utilized advanced AI techniques, including machine learning and climate modeling, to enhance renewable energy systems. The system was designed to:
- Predict Weather Patterns: Use AI-driven climate models to forecast solar irradiance and wind speeds, improving energy generation planning.
- Optimize Energy Output: Apply predictive analytics to adjust solar panel angles and wind turbine operations for maximum efficiency.
Reduce Carbon Footprint: Model and recommend strategies for minimizing energy waste and integrating renewables into the grid, supporting decarbonization efforts.
Development Steps

Data Collection
Gathered data from weather stations, satellite imagery, historical energy production records, and grid usage statistics.

Preprocessing
Cleaned and normalized the data, addressing gaps in weather records and aligning time-series data for analysis.

Model Development
Built machine learning models, such as neural networks and ensemble methods, trained on climate and energy datasets to predict weather and optimize energy systems.

Validation
Tested model accuracy using metrics like mean absolute error for weather predictions and energy output efficiency, validated against real-world performance.

Deployment
Integrated the AI system into the company’s energy management platform, enabling real-time adjustments to renewable energy operations.

Continuous Monitoring & Improvement
Established a feedback loop to refine AI models and adapt to new product categories and store layouts.
Results

Increased Energy Efficiency
AI-driven optimizations boosted solar and wind energy output by 20%, maximizing renewable resource utilization.

Reduced Carbon Emissions
Predictive modeling and grid integration cut carbon emissions by 15%, aligning with sustainability targets.

Improved Grid Reliability
Accurate weather and demand forecasts reduced energy supply disruptions by 25%, enhancing grid stability.

Cost Savings
Operational efficiencies lowered energy production costs by 12%, improving the company’s financial viability.

Scalable Sustainability
The AI framework was adopted across multiple facilities, supporting broader decarbonization efforts and setting a precedent for industry-wide green initiatives.