Case Study
Home » Customer Churn Prediction for Telecom Industry
Customer Churn Prediction for Telecom Industry
Problem Statement
A retail chain faced challenges in managing shelf inventory efficiently. Frequent out-of-stock situations and poor shelf space utilization led to lost sales and decreased customer satisfaction. Traditional manual inventory checks were time-consuming and error-prone, requiring a smarter solution to monitor shelf inventory in real time and optimize product availability.
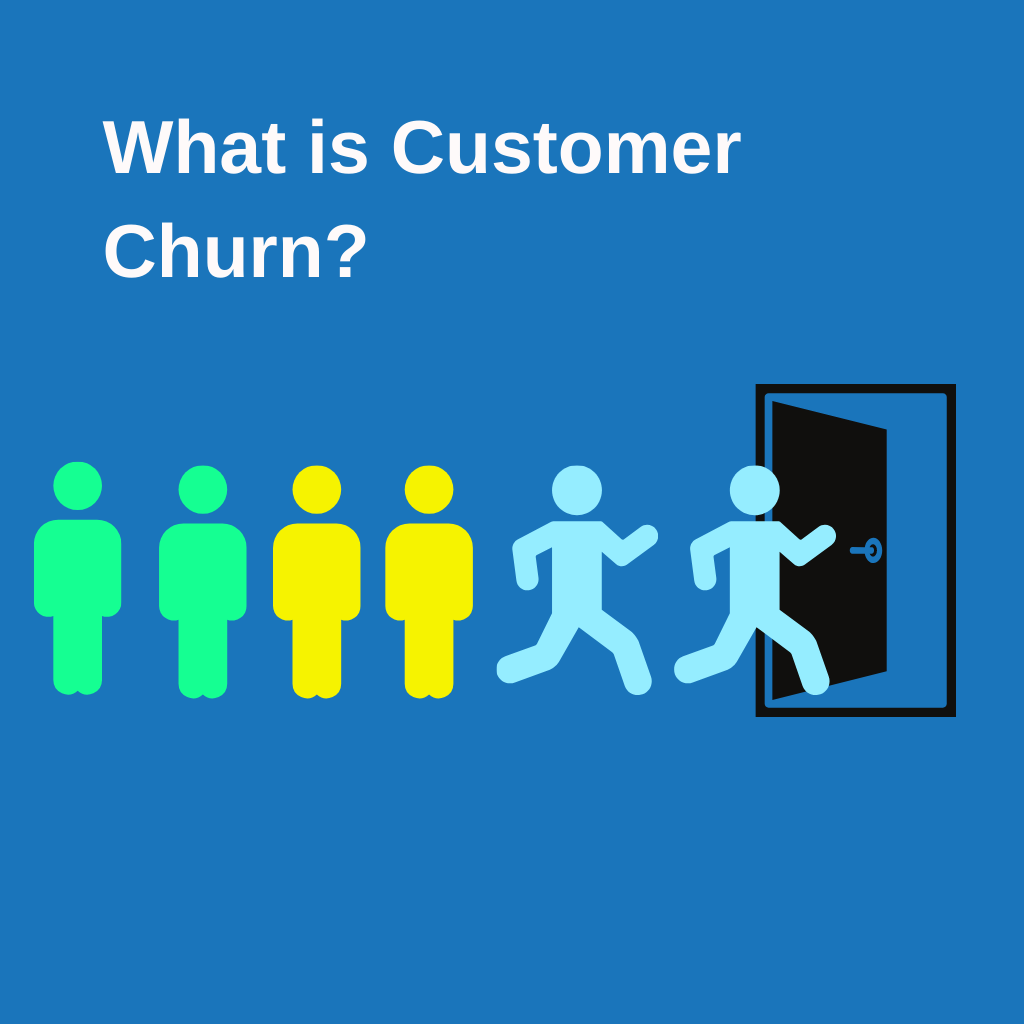
Challenge
The primary challenges in developing a churn prediction model included:
- Data Complexity: Handling large volumes of diverse customer data from various sources, including call records, billing information, and customer service interactions.
- Predictive Accuracy: Developing a model capable of accurately predicting churn to target the right customers with retention strategies.
Operational Integration: Ensuring the churn prediction model could be seamlessly integrated into existing CRM systems for real-time actionability.
Solution Provided
The solution involved the use of advanced machine learning and predictive analytics technologies to analyze customer data and predict churn likelihood. The system was designed to:
- Identify Risk Factors: Analyze customer behavior and service usage patterns to identify potential churn triggers.
- Predict Churn: Employ predictive models to forecast customer churn, enabling proactive retention measures.
- Enhance Customer Engagement: Use insights from the data to tailor communications and offers to improve customer satisfaction and loyalty.
Development Steps

Data Collection
Aggregated and compiled data from multiple sources to create a comprehensive customer database.

Preprocessing
Processed and structured image and sensor data to identify patterns in stock levels and shelf utilization.

Model Development
Developed and trained machine learning models using algorithms like logistic regression and random forests to predict churn likelihood.

Validation
Validated the models through techniques such as cross-validation and performance metrics like accuracy and recall.

Deployment
Integrated the predictive model into the company’s CRM system for real-time churn prediction and management.

Continuous Monitoring & Improvement
Continuously monitored the model’s performance and updated it with new data to adapt to changing customer behaviors and market conditions.
Results

Reduced Out-of-Stock Instances
The predictive model effectively reduced the churn rate by 18%, significantly improving customer retention.

Optimized Shelf Space Utilization
Data-driven insights improved resource allocation, minimizing inefficiencies in service and product offerings

Enhanced Shopping Experience
Personalized retention strategies and proactive engagement led to improved customer satisfaction and loyalty.

Increased Operational Efficiency
Seamless CRM integration enabled automated retention efforts, reducing manual workload and improving response times.

Scalable and Future-Ready Solution
The model was designed for continuous learning and adaptation, ensuring long-term sustainability in a dynamic market.