Case Study
Home » Predictive Maintenance in Manufacturing
Predictive Maintenance in Manufacturing
Problem Statement
A retail chain faced challenges in managing shelf inventory efficiently. Frequent out-of-stock situations and poor shelf space utilization led to lost sales and decreased customer satisfaction. Traditional manual inventory checks were time-consuming and error-prone, requiring a smarter solution to monitor shelf inventory in real time and optimize product availability.
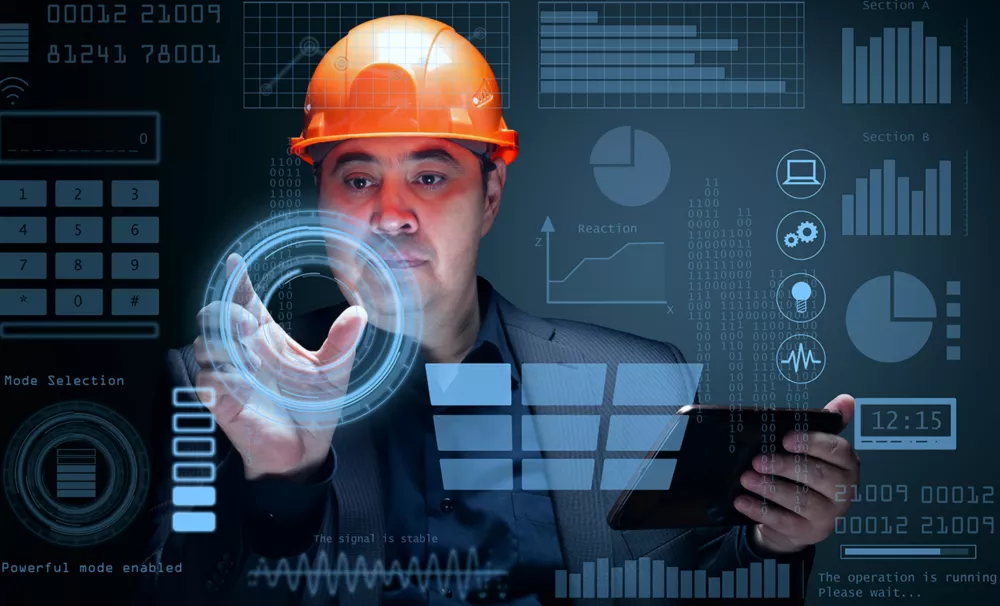
Challenge
Key challenges in implementing a predictive maintenance system included:
- Data Collection and Processing: Gathering real-time data from various sensors installed in heavy machinery.
- Failure Prediction Accuracy: Developing reliable predictive models to accurately forecast machinery failures before they occur.
- Seamless Integration: Ensuring smooth integration of predictive analytics with existing maintenance management systems.
Solution Provided
To address these challenges, an IoT-based predictive maintenance system was deployed using advanced analytics and machine learning. The solution was designed to:
- Monitor Equipment Health: Install IoT sensors on machinery to collect real-time data on temperature, vibration, pressure, and other critical parameters.
- Predict Failures: Use predictive analytics and machine learning algorithms to analyze equipment performance and detect early signs of wear and tear.
Automate Maintenance Scheduling: Optimize maintenance schedules based on predictive insights, reducing unexpected failures and unnecessary maintenance costs
Development Steps

Data Collection
Installed IoT sensors on machinery to collect real-time operational data, including temperature, vibration, and pressure levels.

Preprocessing
Cleaned and standardized sensor data to ensure accuracy in predictive models.

Model Development
Developed predictive analytics models using machine learning algorithms to forecast potential equipment failures.

Validation
Tested the predictive maintenance system in a controlled environment to assess its accuracy in detecting early failure warnings.

Deployment
Integrated the solution into the manufacturing plant’s maintenance management system, enabling automated alerts and scheduled maintenance.

Continuous Monitoring & Improvement
Established real-time monitoring and iterative model improvement to adapt to evolving machinery conditions and optimize accuracy.
Results

Reduced Downtime
The predictive maintenance system significantly minimized unexpected equipment failures, increasing operational efficiency by 20%

Lower Maintenance Costs
Preventive maintenance scheduling reduced unnecessary maintenance activities, optimizing resource allocation.

Enhanced Equipment Lifespan
Early detection of potential issues helped extend machinery life by preventing excessive wear and tear.

Increased Productivity
Improved machinery uptime led to smoother production processes and higher overall efficiency.

Improved Safety
Early failure detection reduced the risk of hazardous breakdowns, ensuring a safer working environment for employees.