Case Study
Home » Smart Agriculture and Crop Monitoring
Smart Agriculture and Crop Monitoring
Problem Statement
A large-scale agricultural enterprise struggled with monitoring crop health across vast farmlands. Traditional methods were labor-intensive and prone to delays in detecting diseases or resource deficiencies, leading to reduced yields and higher operational costs. The enterprise needed an advanced solution to monitor crop health, optimize farming practices, and enhance productivity.
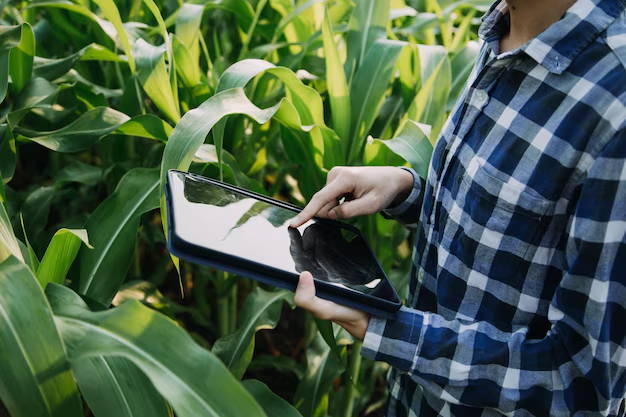
Challenge
Implementing a smart agriculture system presented the following challenges:
- Capturing and processing data from vast and diverse farmland efficiently.
- Detecting early signs of crop diseases and nutrient deficiencies to prevent widespread damage.
Optimizing the use of resources such as water, fertilizers, and pesticides to reduce costs and environmental impact.
Solution Provided
A comprehensive smart agriculture and crop monitoring system was developed using drones equipped with computer vision, IoT sensors, and AI analytics. The solution was designed to:
- Provide aerial imagery of crops to identify health patterns and detect diseases or pests.
- Monitor soil and weather conditions in real-time through IoT sensors.
- Deliver actionable insights for precise resource allocation and farming decisions.
Development Steps

Data Collection
Deployed drones to capture high-resolution images of crops across the farmland. Installed IoT sensors to monitor soil moisture, temperature, humidity, and nutrient levels.

Preprocessing
Processed drone imagery to enhance features such as color, texture, and shape for accurate analysis. Standardized data from IoT sensors to ensure compatibility and reliability.

Model Training
Developed computer vision models to detect crop diseases, pest infestations, and growth anomalies. Built predictive analytics models to forecast optimal planting, irrigation, and harvesting times.

Validation
Tested the system on pilot farmlands to ensure accurate disease detection, resource optimization, and yield prediction.

Deployment
Implemented the solution across the enterprise’s farmland, integrating it with existing farm management systems for seamless operation.

Continuous Monitoring & Improvement
Established a feedback loop to refine models based on new data, improving detection accuracy and predictive capabilities.
Results

Increased Crop Yields
The system improved crop health and productivity, resulting in a 20% increase in overall yields.

Optimized Resource Usage
Precise monitoring and insights reduced water, fertilizer, and pesticide usage, lowering costs and environmental impact.

Early Detection of Crop Diseases
AI-driven disease detection enabled timely interventions, preventing widespread crop loss.

Enhanced Decision-Making
Farmers benefited from data-driven insights, allowing them to make informed decisions about planting, irrigation, and harvesting.

Scalable and Sustainable Solution
The system demonstrated scalability, adapting to various crop types and farm sizes, while supporting sustainable farming practices.